Overcoming Challenges: Implementing AI in Cloud Security
Whatlearned about the company:
Gomboc
Gomboc's AI platform remediates cloud misconfigurations quickly and efficiently with just a few clicks, eliminating the need for ticket-based processes.
impersonates as Cloud Infrastructure Security Consultant
creates article that:
Now compare articles from Tely and other tools:
Overcoming Challenges: Implementing AI in Cloud Security
Overcoming Challenges: Implementing AI in Cloud Security
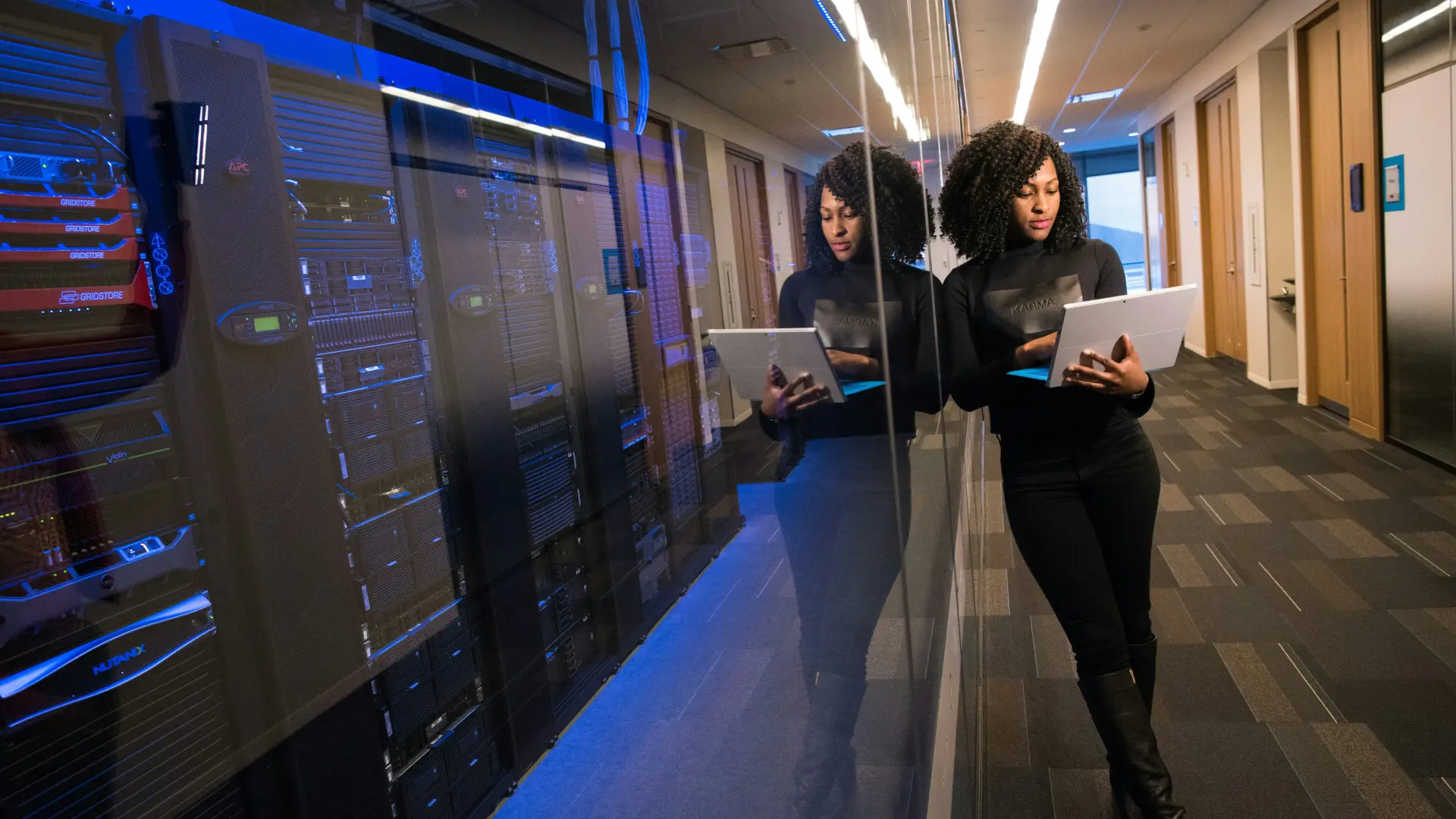
Introduction
Adopting AI in cloud security is pivotal, with over 70% of cloud environments now utilizing AI services. However, this integration comes with its own set of challenges.
This article will explore four key challenges in the intersection of AI and cloud security: technical complexity, ethical considerations, integration with existing systems, and data security and privacy. We will delve into each challenge, discussing the implications and exploring solutions that address these concerns. By understanding these challenges and their potential solutions, organizations can navigate the complexities of AI-driven cloud security and ensure a secure and resilient digital environment.
Challenge 1: Technical Complexity
Adopting AI in cloud security is pivotal, with over 70% of cloud environments now utilizing AI services. Deploying these AI applications requires robust infrastructure to support the significant computational demands.
Organizations must develop comprehensive training and development pipelines to integrate AI effectively. Human experts are critical in managing AI-augmented cloud security, focusing on security and data privacy, including access controls and encryption.
They also validate and test AI-driven features to ensure they align with existing workflows without introducing new vulnerabilities. Gomboc.ai exemplifies this AI-driven approach, offering a deterministic AI engine that remediates cloud security findings and integrates secure fixes directly into the CI/CD pipeline.
This reduces the need for manual remediation and ensures secure infrastructure updates. By focusing on merging AI with practical security solutions, Gomboc.ai enhances collaboration between security and engineering teams and emphasizes the need for strategic partnerships to build a resilient cybersecurity framework.
The platform's integration with various cloud service providers facilitates the configuration of multi-cloud environments, even for teams without prior knowledge. The strategic importance of AI in reinforcing cloud security is underscored by the significant investment in AI-based cybersecurity applications, which reached $265 million in 2019. Success rates for AI-powered cybersecurity tools have been reported at over 80%. With Gomboc.ai's focus on outsmarting threats and 'shifting left' in security practices, the platform supports the proactive stance organizations must take to balance efficiency and security. As we move toward 2024, the year predicted to see a surge in generative AI solutions in security, platforms like Gomboc.ai are set to play a crucial role in enhancing digital trust and revolutionizing cloud security.
Challenge 2: Ethical Considerations
With the rapid adoption of AI-as-a-service, organizations are now grappling with the ethical implications of this technology, particularly in cloud security. Integrating AI into cloud security systems has been instrumental for over a decade, utilizing pattern recognition and anomaly detection to bolster defenses against an increasingly cloud-focused threat landscape.
However, as recent findings from Wiz Research indicate, with AI services present in over 70% of cloud environments, the potential for security risks that could compromise sensitive data and models is significant. Stakeholder engagement is imperative, involving ethicists, policymakers, and the public, to ensure a comprehensive consideration of values and perspectives in AI development.
This is echoed by the commitments made by organizations like D-ID, which adhere to the Partnership on AI's Framework, emphasizing the importance of ethical technology use. AI systems must be transparent to allow users to understand decision-making processes, and datasets require careful scrutiny to prevent discrimination, ensuring fairness in outcomes.
Moreover, 55% of organizations plan to implement generative AI solutions in 2024, signaling a transformative phase in security applications. Despite the enthusiasm for AI's capabilities, concerns about ethical challenges persist, with 42% of executives worried about societal biases in training data and the lack of regulation. PagerDuty reports that more than half of respondents are troubled by disclosing sensitive information and data privacy violations, highlighting the need for continuous monitoring and evaluation of AI systems to address such ethical issues proactively. Ultimately, deploying AI in cloud security necessitates a balance between leveraging its defensive capabilities and upholding ethical standards to foster trust and ensure responsible progress.

Challenge 3: Integration with Existing Systems
Integrating Artificial Intelligence (AI) into cloud security is revolutionizing how organizations monitor and protect their digital ecosystems. A notable example includes a global financial institution that leveraged AI analytics to significantly reduce false positives by more than half while slashing incident response times from hours to mere minutes. Similarly, a healthcare provider integrated AI to safeguard patient data, resulting in a 70% drop in security breaches within a year.
These case studies exemplify AI's transformative impact on cybersecurity, demonstrating its capacity to enhance monitoring capabilities and streamline incident management. However, as the Secure AI Framework (SAIF) suggests, AI deployment must be executed responsibly to avoid potential misuse and vulnerabilities, such as adversarial AI and AI-generated phishing attacks. These risks underscore the necessity of a collaborative approach, where security teams and AI specialists work in tandem to align AI integration with an organization's security needs.
By fostering synergy between data scientists and security personnel and by employing composite AI and threat modeling, organizations can bolster their defenses against cyber threats. The commitment to AI in cybersecurity is further evidenced by the substantial enterprise spending on AI-based applications, which reached $265 million in 2019. Moreover, 44% of organizations have reported an over 80% success rate with AI-powered cybersecurity tools, indicating their growing indispensability in the digital security arsenal.
Embracing AI is not merely about keeping pace but proactively shaping a safer digital future and enhancing productivity across various operational facets. In the evolving landscape of cloud security, platforms like Gomboc AI are at the forefront of innovation, targeting one of the most pressing challenges—remediation backlogs. By integrating its AI engine with infrastructure updates that are pushed directly into the CI/CD pipeline, Gomboc AI automates remediation tasks, thereby reducing the need for extensive human intervention.
This harmonious integration between AI and practical security solutions helps security engineering teams configure multi-cloud environments efficiently without prior specialized knowledge. The deterministic AI engine of Gomboc AI not only addresses compliance issues and cloud misconfigurations but also provides context-aware, secure infrastructure as code IAC solutions. With a vision to reduce remediation backlogs to zero and a commitment to building a resilient cybersecurity framework through strategic partnerships, Gomboc AI contributes to a safer digital environment where DevOps teams can finally remediate their CSPM backlogs with real fixes.
Challenge 4: Data Security and Privacy
The 2023 Thales Cloud Security Study indicates a growing concern for data breaches within cloud infrastructures, with 39% of businesses experiencing such incidents last year. The study highlights human error's significant role, contributing to over half of these breaches.
As businesses acknowledge the sensitivity of their cloud-stored data, the emphasis on stringent access controls and encryption to thwart unauthorized access has never been more critical. Within this context, deploying AI in cloud security presents its own challenges and opportunities.
Gomboc.ai emerges as a solution to the intricacies of AI integration in cloud security. This AI-powered platform is engineered to diminish the remediation backlog effectively, ensuring cloud security findings are addressed promptly.
By leveraging a deterministic AI engine, Gomboc.ai integrates context-aware, [secure infrastructure-as-code IAC directly into the CI/CD pipeline. This integration is crucial for cloud security managers tasked with managing access control and user permissions and maintaining compliance with industry regulations. The platform is a tool for enforcing security measures. It bridges AI advancements and practical security solutions, promoting a culture of safety and innovation within security engineering teams. Gomboc.ai's approach to outsmarting threats by incorporating AI with cloud security and shifting left in the development process exemplifies the proactive stance required to address the complexities of cloud data management. With strategic partnerships and cloud control services, Gomboc.ai and its Chief Product Officer, Matt Sweeney, are revolutionizing [cloud security by blending AI with effective security protocols, enhancing security posture, and fostering digital trust in the cloud ecosystem.

Conclusion
In conclusion, adopting AI in cloud security brings challenges such as technical complexity, ethical considerations, integration with existing systems, and data security and privacy. Organizations need robust infrastructure and human expertise to integrate AI into cloud security effectively. Stakeholder engagement ensures transparency and fairness in decision-making.
Collaboration between security teams and AI specialists is crucial for successful integration. Platforms like Gomboc.ai automate remediation tasks and provide context-aware solutions for efficient multi-cloud configuration. Data security requires stringent access controls and encryption, which can be addressed by platforms like Gomboc.ai that seamlessly integrate secure infrastructure-as-code.
Organizations can navigate the complexities of AI-driven cloud security by understanding these challenges and implementing appropriate solutions. Strategic partnerships and a proactive approach are vital for building a resilient cybersecurity framework that enhances digital trust. With the right tools and practices, organizations can revolutionize cloud security practices, ensuring a safer digital environment for all stakeholders.
Overcoming Challenges: Implementing AI in Cloud Security
Overcoming Challenges: Implementing AI in Cloud Security
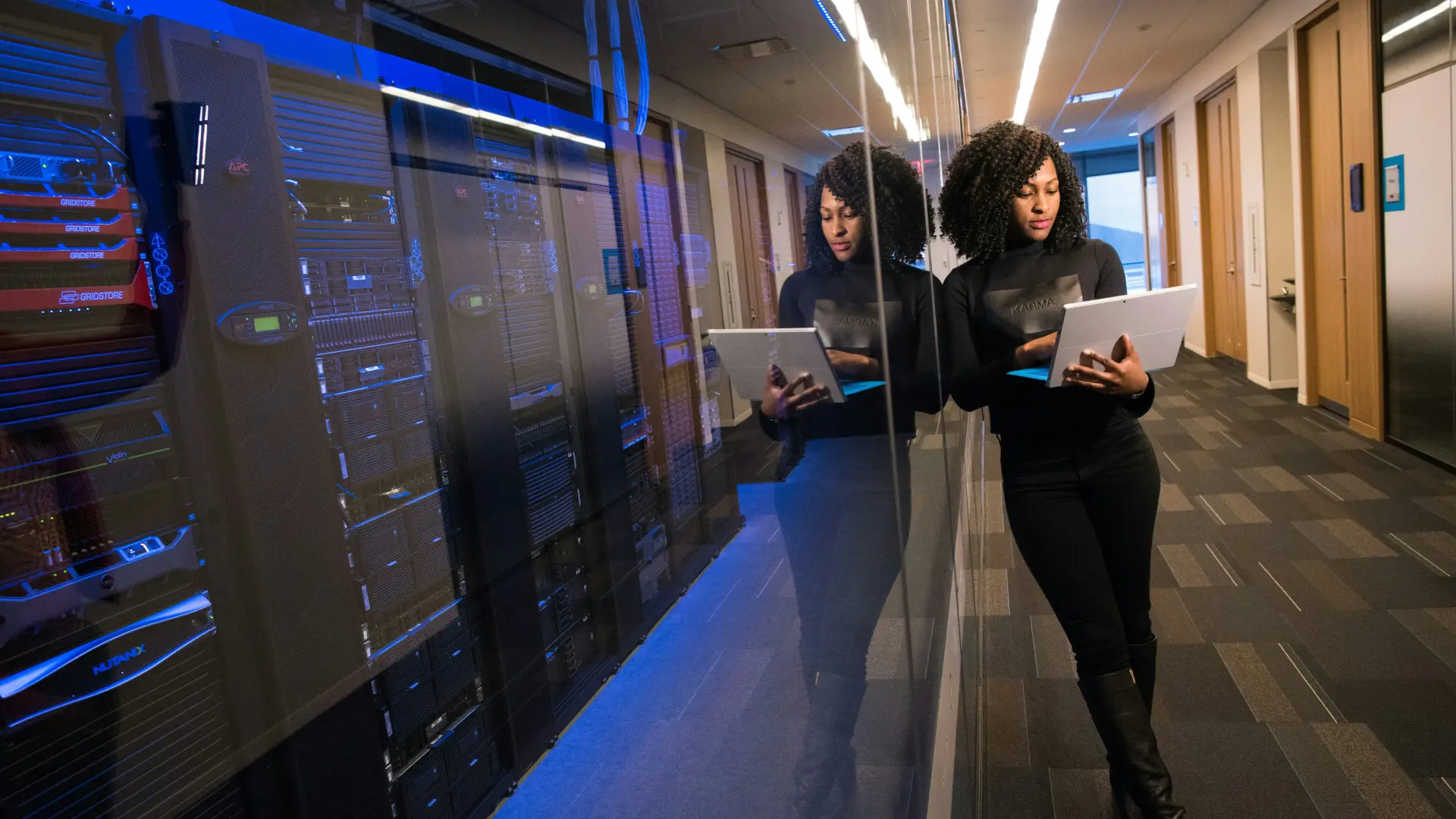
Introduction
In a world where data breaches and cyber threats are growing daily, the role of Artificial Intelligence (AI) in cloud security has never been more crucial. For technology firms, government agencies, and financial services providers, the integration of AI could be a game-changer. However, the road to leveraging AI for cloud security is fraught with challenges. This blog aims to shed light on these hurdles and provide actionable insights on how to overcome them.
The Role of AI in Cloud Security
AI offers remarkable capabilities in identifying and responding to security threats in real time. By analyzing vast amounts of data at lightning speed, AI can detect anomalies that may indicate a security breach. This proactive approach allows organizations to address potential issues before they escalate, thereby minimizing damage and enhancing overall security.
One of the most significant advantages of AI in cloud security is its ability to learn and adapt. Machine learning algorithms can continuously evolve by analyzing new data, improving their accuracy in identifying threats over time. This adaptability is particularly valuable in an environment where cyber threats are constantly changing.
AI also excels in automating responses to security incidents. By using AI, organizations can streamline their security protocols, ensuring that immediate action is taken when a threat is detected. This reduces the reliance on human intervention and allows for faster, more efficient responses to security threats.
Challenges of Implementing AI in Cloud Security
Despite its many benefits, implementing AI in cloud security presents several challenges. One of the most significant hurdles is the technical complexity involved. Developing and deploying AI algorithms requires specialized knowledge and expertise, which may not be readily available within many organizations.
Operational challenges also arise when integrating AI into existing cloud security frameworks. Ensuring that AI systems are compatible with current infrastructure and can work seamlessly with other security measures is essential. This often requires significant time and resources, which can be prohibitive for some organizations.
Ethical concerns are another critical consideration. The use of AI in cloud security raises questions about privacy and data protection. Ensuring that AI systems operate transparently and comply with regulatory requirements is paramount. Failing to address these ethical issues can undermine trust and compromise the effectiveness of AI security measures.
Overcoming the Challenges
To successfully integrate AI into cloud security, organizations must adopt a strategic approach. For technology firms, building a robust AI infrastructure is essential. This involves investing in the necessary hardware and software and recruiting or training personnel with the required expertise.
Government agencies face unique challenges due to the sensitive nature of the data they handle. Ensuring compliance with regulatory requirements and maintaining transparency in AI operations is crucial. Collaborating with private sector experts and other government bodies can help address these challenges and facilitate the successful implementation of AI.
Financial services providers must focus on balancing security with user experience. Implementing AI in cloud security should enhance, not hinder, the user experience. Ensuring that AI systems are user-friendly and do not introduce unnecessary friction is vital. Additionally, financial services providers should prioritize transparency to build trust with their clients.
Case Studies
Several organizations have successfully navigated the challenges of implementing AI in cloud security. One notable example is a leading technology firm that developed a proprietary AI system to monitor and respond to security threats in real time. By investing in the necessary infrastructure and expertise, the company could significantly enhance its security measures and protect its data from potential breaches.
A government agency successfully integrated AI into its cloud security framework by collaborating with private sector experts. This partnership allowed the agency to leverage the latest AI technology while ensuring compliance with regulatory requirements. The result was a more robust and effective security system that protected sensitive government data.
A financial services provider implemented AI to streamline its security protocols and enhance the user experience. By focusing on transparency and user-friendliness, the provider built trust with its clients and improved its overall security posture. This approach demonstrated that it is possible to balance security with user experience effectively.
Future Outlook
The future of AI in cloud security looks promising. Predicted trends include the increased use of AI for predictive analytics, which will allow organizations to anticipate and address threats before they occur. Additionally, advancements in machine learning will continue to improve the accuracy and effectiveness of AI security measures.
Organizations can prepare for these future trends by staying informed about the latest developments in AI and cloud security. Investing in ongoing training and development for personnel is also essential. By staying ahead of the curve, organizations can ensure that they are well-positioned to leverage the full potential of AI in cloud security.
Conclusion
Implementing AI in cloud security presents numerous challenges, but the benefits far outweigh the hurdles. By adopting a strategic approach and addressing technical, operational, and ethical concerns, organizations can successfully integrate AI into their cloud security frameworks.
The examples of successful implementations highlight that overcoming these challenges is possible with the right approach. The future of AI in cloud security is bright, and organizations that stay ahead of the curve will be well-positioned to protect their data and maintain their competitive edge.
Ready to share your experiences or learn more about implementing AI in cloud security? Engage with our community and take the next step in enhancing your organization's security measures.
Overcoming Challenges: Implementing AI in Cloud Security
Overcoming Challenges: Implementing AI in Cloud Security
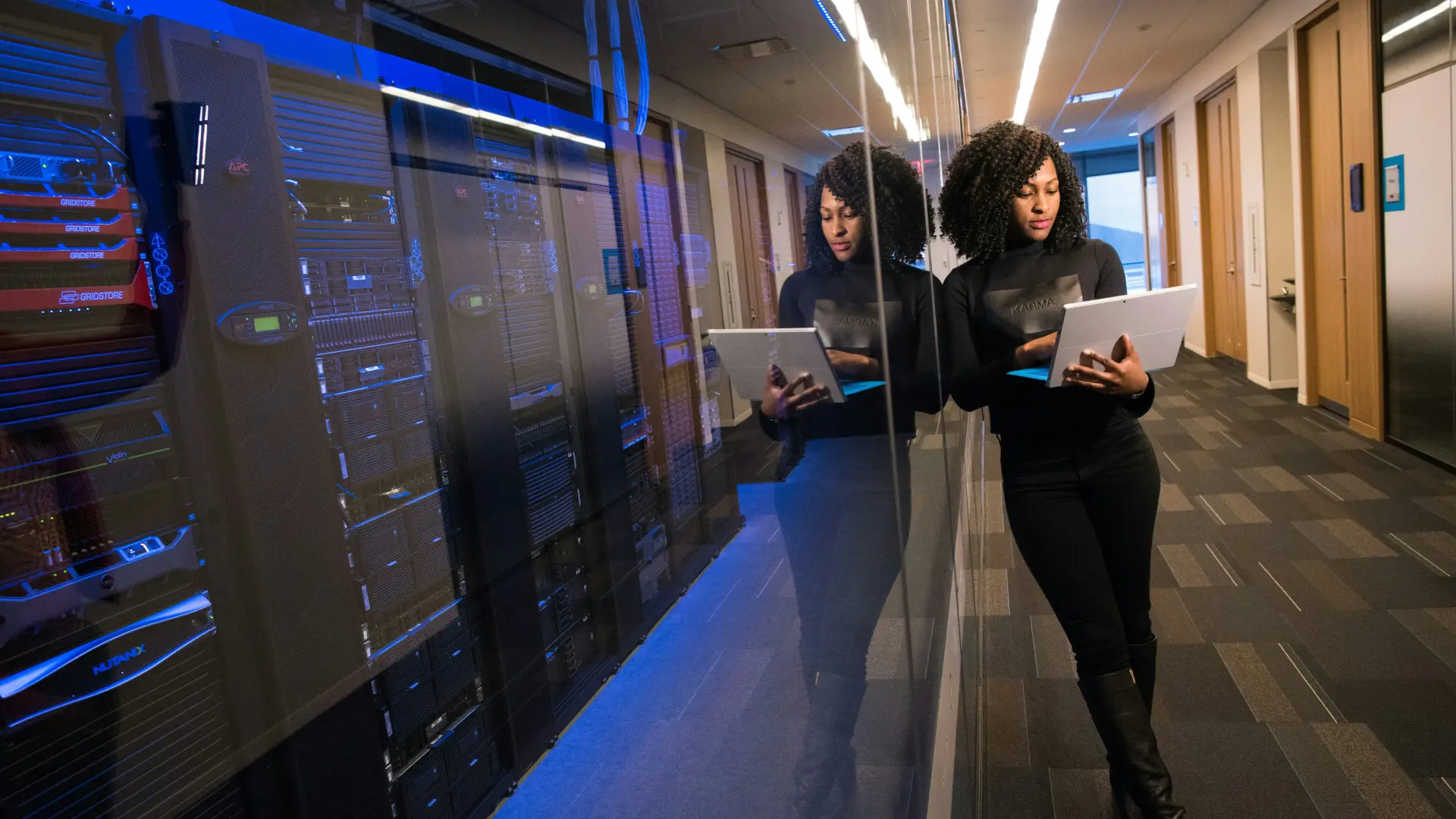
In the realm of cybersecurity, the integration of artificial intelligence (AI) into cloud security measures has emerged as a critical frontier. As organizations increasingly migrate their operations to the cloud, the need for robust security solutions that can adapt and evolve in real-time has become paramount. However, implementing AI in cloud security is not without its challenges. From ensuring data privacy and compliance to addressing the ever-evolving threat landscape, organizations face a myriad of obstacles. This introduction delves into the strategies and best practices for overcoming these challenges and harnessing the power of AI to fortify cloud security defenses. Join us on a journey through the complexities of integrating AI into cloud security and discover how innovative solutions are reshaping the cybersecurity landscape.
Challenges in Implementing AI in Cloud Security
Data Privacy Concerns
As organizations increasingly rely on cloud services, ensuring data privacy becomes a critical challenge. AI algorithms need access to vast amounts of data to learn and make informed decisions, raising concerns about data privacy and compliance with regulations like GDPR. Organizations must implement robust encryption methods, access controls, and data anonymization techniques to protect sensitive information.
Integration Complexities
Integrating AI capabilities into existing cloud security infrastructure can be complex. Ensuring seamless integration, compatibility with existing tools, and minimal disruption to operations are key challenges faced by organizations. Additionally, organizations need to consider the scalability of AI solutions to meet the dynamic nature of cloud environments and the diverse needs of different applications.
Skill Gap in AI Expertise
Another significant challenge is the shortage of professionals with expertise in both AI and cloud security. Building and maintaining AI-powered security systems require specialized skills that are currently in high demand but short supply. Organizations can address this challenge by investing in training programs, collaborating with educational institutions, and fostering a culture of continuous learning within their teams. Furthermore, leveraging AI technologies to automate routine security tasks can help bridge the skill gap and enhance overall security posture.
Regulatory Compliance Challenges
Complying with data protection regulations and industry standards adds another layer of complexity to implementing AI in cloud security. Organizations must navigate a complex landscape of regulations such as HIPAA, PCI DSS, and CCPA while ensuring that AI systems adhere to ethical guidelines and do not perpetuate biases or discrimination. Implementing transparent AI algorithms and conducting regular audits are essential to demonstrate compliance and build trust with stakeholders.
Continuous Monitoring and Adaptation
Maintaining the effectiveness of AI-powered security measures requires continuous monitoring and adaptation. Threat actors are constantly evolving their tactics, necessitating proactive measures to detect and respond to emerging threats. Organizations need to establish robust monitoring mechanisms, leverage threat intelligence feeds, and conduct regular security assessments to identify vulnerabilities and enhance their defense mechanisms.
Conclusion
Overcoming the challenges in implementing AI in cloud security requires a holistic approach that addresses technical, organizational, and regulatory aspects. By prioritizing data privacy, investing in talent development, ensuring regulatory compliance, and fostering a culture of innovation, organizations can harness the full potential of AI to enhance their cloud security posture and protect their digital assets.
Strategies to Overcome Challenges
Leveraging Enhanced Data Encryption Techniques
In the era of digital transformation, ensuring robust data security is paramount for organizations. Enhanced data encryption techniques play a crucial role in safeguarding sensitive information from cyber threats and unauthorized access. By employing cutting-edge encryption algorithms, multi-factor authentication, and secure communication protocols, businesses can fortify their data protection measures and mitigate potential risks effectively. Moreover, implementing end-to-end encryption across all communication channels and data storage systems enhances confidentiality and integrity, ensuring compliance with stringent data privacy regulations.
Harnessing Automated AI Integration Tools
The integration of Artificial Intelligence (AI) technologies has redefined operational efficiency and decision-making processes across industries. Automated AI integration tools empower enterprises to automate mundane tasks, analyze vast datasets swiftly, and derive actionable insights for strategic planning. By leveraging machine learning algorithms and predictive analytics, businesses can enhance operational agility, optimize resource allocation, and drive sustainable growth. Additionally, deploying AI-powered cybersecurity solutions enhances threat detection capabilities, proactively identifying and mitigating security vulnerabilities to safeguard critical assets and maintain business continuity.
Fostering a Culture of Continuous Learning through Training Programs
In a rapidly evolving technological landscape, empowering employees with the requisite skills and knowledge is imperative for organizational success. Implementing comprehensive training programs tailored to upskill employees not only enhances their competencies but also cultivates a culture of continuous learning within the workforce. By offering specialized workshops, online courses, and hands-on training sessions, companies can nurture talent, foster innovation, and adapt proactively to industry disruptions. Furthermore, establishing mentorship programs and knowledge-sharing platforms fosters collaboration and knowledge transfer, fostering a culture of continuous improvement and professional development.
Embracing Agile Practices for Adaptive Resilience
Adopting agile methodologies and practices is essential for organizations seeking to navigate unforeseen challenges and market uncertainties effectively. By embracing iterative development cycles, cross-functional collaboration, and rapid prototyping, businesses can enhance their adaptive resilience and responsiveness to changing market dynamics. Agile frameworks such as Scrum and Kanban enable teams to prioritize tasks, iterate on feedback, and deliver value incrementally, fostering a culture of innovation and continuous improvement. Additionally, implementing DevOps practices streamlines development and operations, promoting seamless integration, continuous delivery, and rapid response to changing business requirements.
Cultivating Strategic Partnerships for Synergistic Growth
Collaborating with strategic partners and industry stakeholders can amplify organizational capabilities, drive innovation, and unlock new growth opportunities. By forging symbiotic relationships with complementary businesses, technology providers, and research institutions, companies can leverage collective expertise, resources, and market insights to co-create innovative solutions, expand market reach, and accelerate digital transformation initiatives. Moreover, establishing strategic alliances for joint research and development initiatives fosters knowledge exchange, accelerates product innovation, and enhances competitive advantage in dynamic market landscapes.
Case Studies
- Successful Implementation of AI in Cloud Security
In this case study, we explore a real-world scenario where Artificial Intelligence (AI) was successfully implemented in cloud security. The case study delves into the specific challenges faced by the organization, the innovative strategies employed to integrate AI, and the remarkable outcomes achieved. By examining this successful implementation, readers can gain valuable insights into the benefits of leveraging AI for enhancing cloud security measures.
- Lessons Learned from Failed AI Integration Projects
In this case study, we analyze a failed AI integration project to extract crucial lessons and insights. By examining the reasons behind the failure, the critical mistakes made during the implementation phase, and the overall implications of the unsuccessful integration, readers can understand the importance of proper planning and execution in AI projects. Learning from the pitfalls and challenges faced in this case study can help organizations avoid common mistakes and pitfalls in their own AI integration endeavors.
- Impact of AI on Cybersecurity
Beyond the specific case studies, it is essential to discuss the broader impact of AI on cybersecurity. AI technologies have revolutionized the way organizations detect, prevent, and respond to cyber threats. By leveraging machine learning algorithms and predictive analytics, AI can enhance threat intelligence, automate security operations, and strengthen overall cybersecurity posture. Understanding the transformative role of AI in cybersecurity is crucial for organizations looking to stay ahead of evolving cyber threats.
- Future Trends in AI-driven Security Solutions
Looking ahead, the future of AI-driven security solutions holds immense potential for innovation and advancement. From predictive threat modeling to autonomous incident response, AI is poised to reshape the cybersecurity landscape. Exploring upcoming trends such as AI-powered threat hunting, adaptive security architectures, and AI-driven risk assessment can provide valuable insights into the evolving nature of cybersecurity defenses.
These case studies and discussions underscore the critical role of AI in shaping the future of cloud security and cybersecurity. By learning from both successful implementations and failed integration projects, organizations can chart a strategic path towards harnessing the full potential of AI for safeguarding digital assets and mitigating cyber risks.
Search engines index & rank higher Tely’s expert-level articles
For each article we use at least 30 sources to add cases, report data, quotes and infographics.
Tely AI uses real world information to position your company as an industry expert
Tely AI generates infographics to capture visitors attention
Tely inserts quotes and expert opinions of niche industry leaders
Tely paraphrases all texts to sound human and bypass AI content detectors
As we reach 2023, a GPT-4-based model has solved 84.3% of problems, nearing the human baseline of 90%. As we continue to push AI's limits in mathematics, we are compelled to create new benchmarks to highlight the differences and advantages between human and AI problem-solving.
In a test involving 30 problems from the International Mathematical Olympiad, AlphaGeometry was able to solve 25. This performance significantly surpasses previous methods, which could only solve 10 problems.
The ability of AI to process and analyze massive data sets has the potential to revolutionize the methodologies and problem-solving approaches used in mathematics.
“Machine learning tools are very good at recognizing patterns and analyzing very complex problems.”
Consider the “ai math” problem, a complex mathematical challenge that has been made more manageable through AI's capabilities. This not only saves considerable time but also opens new avenues for innovative mathematical research.